Merve Noyan PRO
AI & ML interests
Articles
Organizations
merve's activity
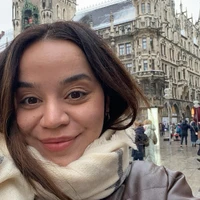
🔖 models: https://huggingface.co/PekingU
🔖 demo: merve/RT-DETR-tracking-coco
📝 paper: DETRs Beat YOLOs on Real-time Object Detection (2304.08069)
📖 notebook: https://github.com/merveenoyan/example_notebooks/blob/main/RT_DETR_Notebook.ipynb
YOLO models are known to be super fast for real-time computer vision, but they have a downside with being volatile to NMS 🥲
Transformer-based models on the other hand are computationally not as efficient 🥲
Isn't there something in between? Enter RT-DETR!
The authors combined CNN backbone, multi-stage hybrid decoder (combining convs and attn) with a transformer decoder. In the paper, authors also claim one can adjust speed by changing decoder layers without retraining altogether.
The authors find out that the model performs better in terms of speed and accuracy compared to the previous state-of-the-art. 🤩
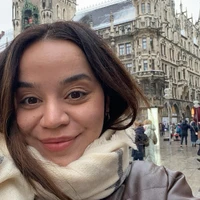
Learn about more machine learning tasks at https://huggingface.co/tasks
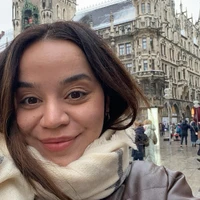
Today we release a notebook and a walkthrough blog on fine-tuning Florence-2 on DocVQA dataset @andito @SkalskiP
Blog: https://huggingface.co/blog 📕
Notebook: https://colab.research.google.com/drive/1hKDrJ5AH_o7I95PtZ9__VlCTNAo1Gjpf?usp=sharing 📖
Florence-2 is a great vision-language model thanks to it's massive dataset and small size!
This model requires conditioning through task prefixes and it's not as generalist, requiring fine-tuning on a new task, such as DocVQA 📝
We have fine-tuned the model on A100 (and one can also use a smaller GPU with smaller batch size) and saw that model picks up new tasks 🥹
See below how it looks like before and after FT 🤩
Play with the demo here andito/Florence-2-DocVQA 🏄♀️
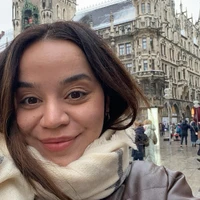
4M is a multimodal training framework introduced by Apple and EPFL.
Resulting model takes image and text and output image and text 🤩
Models: EPFL-VILAB/4m-models-660193abe3faf4b4d98a2742
Demo: EPFL-VILAB/4M
Paper: 4M-21: An Any-to-Any Vision Model for Tens of Tasks and Modalities (2406.09406)
This model consists of transformer encoder and decoder, where the key to multimodality lies in input and output data:
input and output tokens are decoded to generate bounding boxes, generated image's pixels, captions and more!
This model also learnt to generate canny maps, SAM edges and other things for steerable text-to-image generation 🖼️
The authors only added image-to-all capabilities for the demo, but you can try to use this model for text-to-image generation as well ☺️
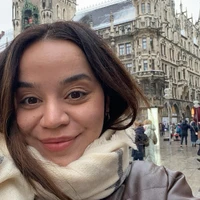
Demo 👉🏻 gokaygokay/Florence-2
Collection 👉🏻 microsoft/florence-6669f44df0d87d9c3bfb76de
This model can handle tasks that vary from OCR to semantic segmentation.
The difference from previous models is that the authors have compiled a dataset consisting of 126M images with 5.4B annotations labelled with their own data engine pseudolabelled by smaller specialized models and APIs.
The model has a similar architecture to previous models: an image encoder and a multimodality encoder with a text decoder. The authors have compiled the multitask dataset with prompts for each task.
You can also fine-tune this model on any task of choice. The authors also released different results on downstream tasks and reported their results when un/freezing the vision encoder 🤓📉
They have released fine-tuned models too, you can find them in the collection above 🤗
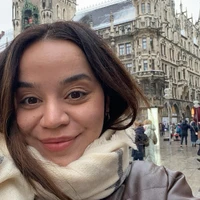
PixelProse is a captioning dataset of 16M image-caption pairs, with less toxicity and higher details ✨
tomg-group-umd/pixelprose
The existing suite of captioning datasets consists of web scrapes that have alt text that is either irrelevant or not descriptive. The authors of this paper have taken those datasets, filtered for CSAM, passed it with a prompt to Gemini Vision Pro. They also removed PII and detoxified the resulting dataset.
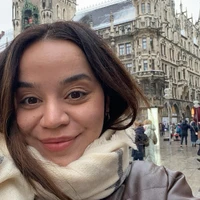
It’s Depth Anything, but scaled with both larger teacher model and a gigantic dataset!
Here's a small TLDR of paper with a lot of findings, experiments and more.
I have also created a collection that has the models, the dataset, the demo and CoreML converted model 😚 merve/depth-anything-v2-release-6671902e798cd404513ffbf5
The authors have analyzed Marigold, a diffusion based model against Depth Anything and found out what’s up with using synthetic images vs real images for MDE:
🔖 Real data has a lot of label noise, inaccurate depth maps (caused by depth sensors missing transparent objects etc) and there are many details overlooked
🔖 Synthetic data have more precise and detailed depth labels and they are truly ground-truth, but there’s a distribution shift between real and synthetic images, and they have restricted scene coverage
The authors train different image encoders only on synthetic images and find out unless the encoder is very large the model can’t generalize well (but large models generalize inherently anyway) 🧐
But they still fail encountering real images that have wide distribution in labels (e.g. diverse instances of objects) 🥲
Depth Anything v2 framework is to..
🦖 Train a teacher model based on DINOv2-G based on 595K synthetic images
🏷️ Label 62M real images using teacher model
🦕 Train a student model using the real images labelled by teacher
Result: 10x faster and more accurate than Marigold!
The authors also construct a new benchmark called DA-2K that is less noisy, highly detailed and more diverse!
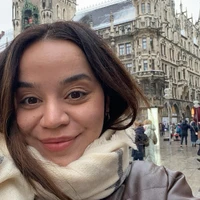
Have you claimed your papers and linked your models/datasets/demos?
This will increase visibility and impact of your paper 💫
To index your papers, go here
CVPR2024/CVPR2024-papers
Find your paper, click on paper page link, index the paper, then click on your name (workflow is below 👇🏻)
If you'd like to add links to your paper, go here CVPR2024/update-CVPR2024-papers
login, find your paper's id, retrieve the paper, fill in the info and submit!
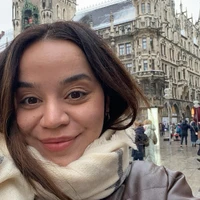
A repository with notebooks on shrinking, optimizing, speeding-up, customizing large vision models! https://github.com/merveenoyan/smol-vision
thank you for all you do for good open-source <3
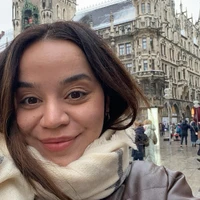
I asked it to describe my favorite Howl's Moving Castle scene and here's how it went 👇🏻
joke aside it seems to outperform the previous VLMs. however the license isn't open-source 📈
model repo: THUDM/glm-4v-9b
a community member has built a demo: vilarin/VL-Chatbox
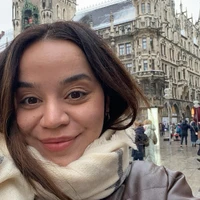
LLaVA 1.6 is outperforming proprietary VLMs, making it a very robust choice for production!
It is now hosted as a leaderboard MM-UPD/MM-UPD_Leaderboard 🏆💕
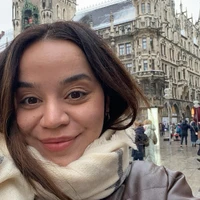
@hakunamatata1997 why not use a document LM though if you were to combine OCR and VLM, if you do the latter it will for sure perform worse because you're missing out a lot on the layout, chart etc anyway. maybe try this https://huggingface.co/spaces/mPLUG/DocOwl it's very good
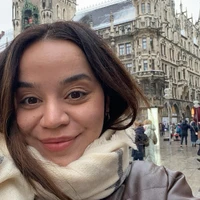
Hello
@anothercoder2
interesting, can you see the files through the CLI though? is this your local setup? I think you need to find the correct path inside /downloads and give load_from_disk
that. because many datasets are cached in same folder it needs the exact path. (which often is a folder under ~/.cache/huggingface/datasets/downloads
with a unique ID assigned)
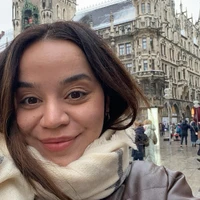
A new paper (by @HuanjinYao et al) built a dense connector that does it better! HuanjinYao/DenseConnector-v1.5-8B
HuanjinYao/denseconnector-66500e173fc8c9f05dc98dea
VLMs consist of an image encoder block, a projection layer that projects image embeddings to text embedding space and then a text decoder sequentially connected 📖
This paper explores using intermediate states of image encoder and not a single output 🤩
The authors explore three different ways of instantiating dense connector: sparse token integration, sparse channel integration and dense channel integration. (see paper on how they do it Dense Connector for MLLMs (2405.13800))
They explore all three of them integrated to LLaVA 1.5 and found out each of the new models are superior to the original LLaVA 1.5 🥹 I tried the model and it seems to work very well. As part of the release, the authors have released various ckpts based on different decoders (Vicuna 7/13B and Llama 3-8B) that you can find in the collection 🤗
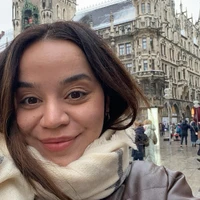
you can use Colab's instances to do QLoRA FT, and then for Space we will give ZeroGPU :)
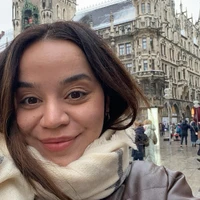
you can use Colab's instances to do QLoRA FT, and then for Space we will give ZeroGPU :)
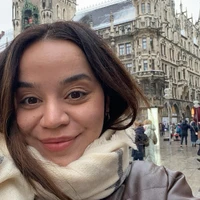
You can pick any dataset of your choice!
Example code: https://colab.research.google.com/drive/1x_OEphRK0H97DqqxEyiMewqsTiLD_Xmi?usp=sharing (you can use a lower GPU with QLoRA)
Datasets:
https://huggingface.co/datasets?task_categories=task_categories:text-to-image&sort=trending
https://huggingface.co/datasets?task_categories=task_categories:image-to-text&sort=trending
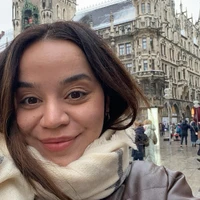
@HakunaMatata1997
hello!
I think on top of my head I can't think of an OCR model specifically, I was mostly using easyocr. OCR is a problem that is pretty much solved, so most of the AI work around docs are focused on understanding documents (because it's more than image -> text, it involves text, charts, tables, whole layout and more)
if you really want OCR there are models like https://huggingface.co/facebook/nougat-base that is for PDF to markdown for instance.
I can also recommend some for document understanding in general (which works on text + chart + image + layout) zero shot or as a backbone to finetune.
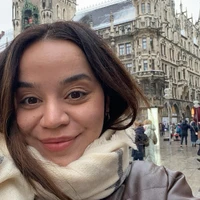
for instance, if you want to collaborate with an external organization you don't want to use your write token since they can access everything you can access. instead you can set token access to repositories under that org only like below
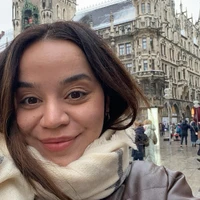
merve/paligemma-doc
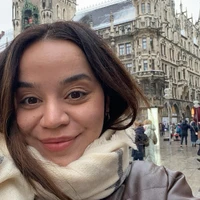
@Cuiunbo ah yes, right. these type of models are "OCR free" meaning it understands and responds the image and not uses an extra ocr on them per se. those datasets are also ocr free I think. good thing about ocr free approach is that features like layout, charts, tables etc are also understood. maybe try prompts to do purely ocr? high res works well also on handwritings etc
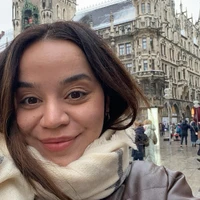
Here's the notebook to do so: https://colab.research.google.com/drive/16-Tq-iAMHNlSjDWgz43kYDMJERjU_KHW?usp=sharing 🤗
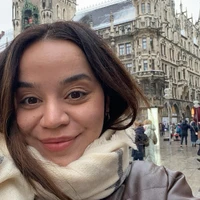
@Cuiunbo I think in model card you can see OCR (document understanding in general) fine-tuned model with associated benchmark on test dataset
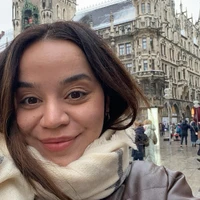
CuMo is a new vision language model that has MoE in every step of the VLM (image encoder, MLP and text decoder) and uses Mistral-7B for the decoder part 🤓
You can try it yourself here: shi-labs/CuMo-7b-zero
the authors firstly did pre-training of MLP with the by freezing the image encoder and text decoder, then they warmup the whole network by unfreezing and finetuning which they state to stabilize the visual instruction tuning when bringing in the experts. 🤓
the mixture of experts MLP blocks above are simply the same MLP blocks initialized from the single MLP that was trained during pre-training and fine-tuned in pre-finetuning.
it works very well (also tested myself) that it outperforms the previous sota of it's size LLaVA NeXt and IDEFICS2-8B in several benchmarks! 😍
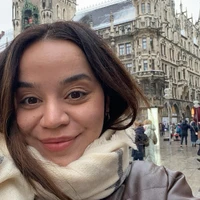
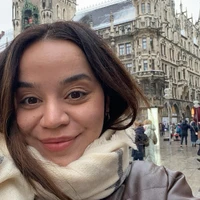
@MoonRide if you check the model card you can see the scores. mix models are trained on a mix of academic benchmark datasets (coco captions, vqav2, ocrvqa etc), where you just say e.g. "caption" and it captions. these datasets often have shorter descriptions and not long prompts, however they're grounded so they are good in the test sets of those benchmarks and can be used in many industry use cases (document AI etc since it hardly hallucinates). for your prompt, I just input "caption" and it came up with very grounded caption for instance.
the main point of PaliGemma release is to release finetuneable models, not provide heavy models with wide zero shot capabilities (where you input super long instruction or chat like prompts) so if you want, you can finetune a "pt" model on any benchmark of your choice and it should perform well.
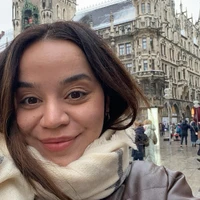
@MoonRide it's not about benchmarks, but the training dataset of the mix checkpoint is different than your use case. I responded on your issue with more details.
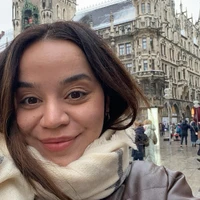
📝 Comes in 3B, pretrained, mix and fine-tuned models in 224, 448 and 896 resolution
🧩 Combination of Gemma 2B LLM and SigLIP image encoder
🤗 Supported in transformers
PaliGemma can do..
🧩 Image segmentation and detection! 🤯
📑 Detailed document understanding and reasoning
🙋 Visual question answering, captioning and any other VLM task!
Read our blog 🔖 hf.co/blog/paligemma
Try the demo 🪀 hf.co/spaces/google/paligemma
Check out the Spaces and the models all in the collection 📚 google/paligemma-release-6643a9ffbf57de2ae0448dda
Collection of fine-tuned PaliGemma models google/paligemma-ft-models-6643b03efb769dad650d2dda
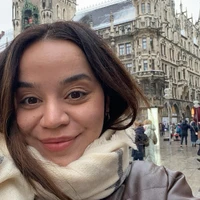
BLINK: evaluates tasks that humans can solve within a blink 👀 BLINK-Benchmark/BLINK
SEED-2-Plus: multichoice questions on charts, maps, webs 😍 AILab-CVC/SEED-Bench-2-plus
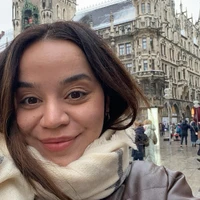
Try them yourself here merve/compare_VLMs
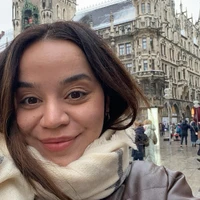
Hiya, are you planning to open-source the models?
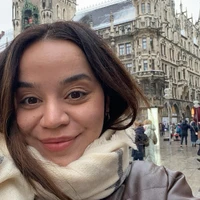
As of now, most VLMs, including GPT-4V and LLaVA-Next-34B, struggle with refusing to answer
Dataset MM-UPD/MM-UPD
Paper Unsolvable Problem Detection: Evaluating Trustworthiness of Vision Language Models (2403.20331)
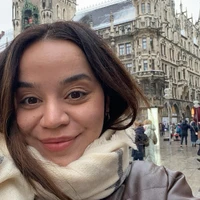
I have seen many open-source document models, and I am amazed by what IDEFICS2 has done with document understanding 🤯🤩 it's not something you've ever seen before! HuggingFaceM4/idefics-8b
Please use it! Has Apache 2.0 license ❤️
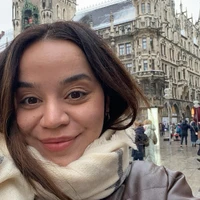
This checkpoint is not optimized to chat, but rather works very well for various tasks, incl visual question answering and document tasks 💬📑
Chatty one is coming soon!
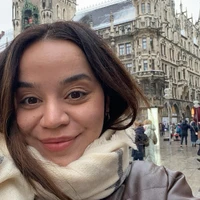
It comes with the last release of transformers 🎁 Demo and more in this post!
SegGPT is an extension of the Painter, where you speak to images with images: the model takes in an image prompt, transformed version of the image prompt, the actual image you want to see the same transform, and expected to output the transformed image.
SegGPT consists of a vanilla ViT with a decoder on top (linear, conv, linear).
The model is trained on diverse segmentation examples, where they provide example image-mask pairs, the actual input to be segmented, and the decoder head learns to reconstruct the mask output.
This generalizes pretty well!
The authors do not claim state-of-the-art results as the model is mainly used zero-shot and few-shot inference. They also do prompt tuning, where they freeze the parameters of the model and only optimize the image tensor (the input context).
Thanks to 🤗 transformers you can use this model easily!
See here https://huggingface.co/docs/transformers/en/model_doc/seggpt
I have built an app for you to try it out. I combined SegGPT with Depth Anything Model, so you don't have to upload image mask prompts in your prompt pair 🤗
Try it here merve/seggpt-depth-anything
Also check out the collection merve/seggpt-660466a303bc3cd7559d271b
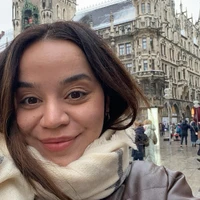
I think it would be nice if we could have what the data looks like in the tl;dr, how it was curated, the license, what type of model it can be trained with and so on, it would be very useful for me 🤩
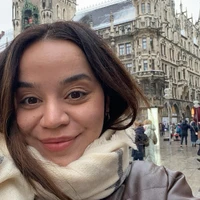
Demo: merve/llava-next
Notebook: https://colab.research.google.com/drive/1afNudu72SNWZCYtCVrRlb9T9Vj9CFJEK?usp=sharing
LLaVA is essentially a vision-language model that consists of ViT-based CLIP encoder, a MLP projection and Vicuna as decoder ✨
LLaVA 1.5 was released with Vicuna, but LLaVA NeXT (1.6) is released with four different LLMs:
- Nous-Hermes-Yi-34B
- Mistral-7B
- Vicuna 7B & 13B
Mistral and Nous-Hermes-Yi-34B are performing better and have better commercial use.
Moreover, according to authors' findings, the improvements comes from more diverse and high quality data mixture and dynamic high resolution.
LLaVA based on Nous-Hermes-Yi-34B outperforms many other models, including Gemini in various multimodal understanding and generation benchmarks 😊
I really like your work, and I did check moondream GH repository. Was wondering if you'd like to share your training details and findings on aligning text decoder and vision encoder and projection layer.
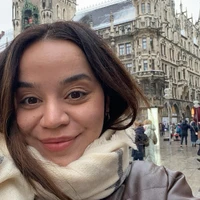
My favorite is KOSMOS-2, because it's a grounded model (it doesn't hallucinate).
In this demo you can,
- ask a question about the image,
- do detailed/brief captioning,
- localize the objects! 🤯
It's just amazing for VLM to return bounding boxes 🤩
Try it here merve/kosmos2
one of the best research questions I've seen recently 😊
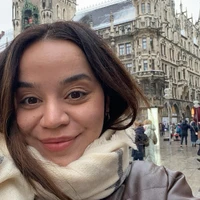
UDOP by MSFT is a bleeding-edge model that is capable of many tasks, including question answering, document editing and more! 🤯
Demo 👉 merve/UDOP
It is a model that combines vision, text and layout. 📝
This model is very interesting because the input representation truly captures the nature of the document modality: text, where the text is, and the layout of the document matters!
If you know T5, it resembles that: it's pre-trained on both self-supervised and supervised objectives over text, image and layout.
To switch between tasks, one simply needs to change the task specific prompt at the beginning, e.g. for QA, one prepends with Question answering.
As for the architecture, it's like T5, except it has a single encoder that takes in text, image and layout, and two decoders (text-layout and vision decoders) combined into one.
The vision decoder is a masked autoencoder (thus the capabilities of document editing).
For me, the most interesting capability is document reconstruction, document editing and layout re-arrangement. This decoder isn't released though because it could be used maliciously to fake document editing.
Overall, the model performs very well on document understanding benchmark (DUE) and also information extraction (FUNSD, CORD) and classification (RVL-CDIP) for vision, text, layout modalities.
You can learn more about the model from below resources (h/t to
@nielsr ), thanks a lot for reading 🤗
Docs: https://huggingface.co/docs/transformers/main/en/model_doc/udop 📚
Checkpoints: microsoft/udop-65e625124aee97415b88b513
Demo notebooks: https://github.com/NielsRogge/Transformers-Tutorials/tree/master/UDOP 📕
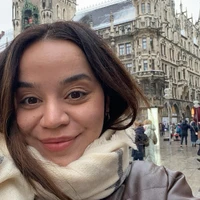
as usual trained on lego dataset I compiled, I compared them with previously trained pivotal tuned model and the normal DreamBooth model before that 😊
Notebook by @linoyts https://colab.research.google.com/drive/134mt7bCMKtCYyYzETfEGKXT1J6J50ydT?usp=sharing
Integration to PEFT by @BenjaminB https://github.com/huggingface/peft/pull/1474 (more info in the PR)
Thanks a lot for the blog post, it's very informative 🤗
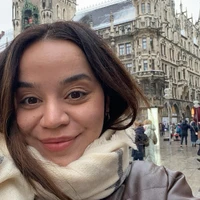
The models are ranked based on ELO, you can rate the responses to preselected examples or try with your input 🤗
WildVision/vision-arena
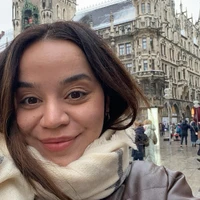
thanks a lot for sharing 🤗 also cc @reach-vb
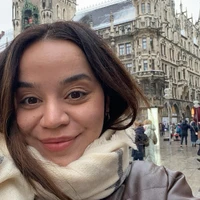
their secret sauce is.. synthetic data pseudolabeled by Stockfish engine 😀
2024 really is the year of synthetic data across all domains!
There's a nice discussion here, join us Grandmaster-Level Chess Without Search (2402.04494)
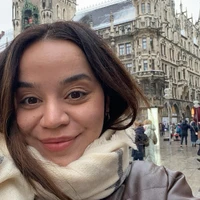
Both of these pages consists of models you can use off-the-shelf 💜
Reading these you can start building in no time 🛠️
Task page for zero-shot object detection 👉 https://huggingface.co/tasks/zero-shot-object-detection 📚
Task page for mask generation 👉 https://huggingface.co/tasks/mask-generation 📖
➕ for comment upvotes or ⬆️
What is the limitation that you haven't use your own EVACLIP here?
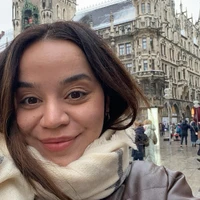
The new SotA CLIP-like model 🏆
Highlights ✨
- Performs better in linear probing
- Outperforms in Zero-Shot Image-Text Retrieval
- Higher zero-shot accuracy in IN-1K
As usual, try it with the notebook I built for you https://colab.research.google.com/drive/1K7DdCORC3x4qyhwhuB4fT4wcfJ_BQLKw?usp=sharing#scrollTo=0ZS_lJ7SK6Ys
I also built a Space for you to compare the output probabilities to CLIP, seems that EVACLIP is more "sure" of it's results 😊 merve/EVACLIP
The authors have shared 8B checkpoints open with Apache 2.0 license 💜 and it's built on top of transformers, super easy to use! BAAI/EVA-CLIP-8B
Read the paper EVA-CLIP-18B: Scaling CLIP to 18 Billion Parameters (2402.04252) 📄
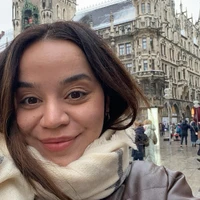
Before we begin: Depth Anything is recently integrated to 🤗 transformers and you can use it with three lines of code! ✨
from transformers import pipeline
pipe = pipeline(task="depth-estimation", model="LiheYoung/depth-anything-small-hf")
depth = pipe(image)["depth"]
We have also built an app for you to compare different depth estimation models 🐝 🌸 merve/compare_depth_models
Check out Depth Anything in Web by @Xenova Xenova/depth-anything-web
The model's success heavily depends on unlocking the use of unlabeled datasets, although initially the authors used self-training and failed.
What the authors have done:
➰ Train a teacher model on labelled dataset
➰ Guide the student using teacher and also use unlabelled datasets pseudolabelled by the teacher
However, this was the cause of the failure, as both architectures were similar, the outputs were the same.
So the authors have added a more difficult optimization target for student to learn additional knowledge on unlabeled images that went through color jittering, distortions, Gaussian blurring and spatial distortion, so it can learn more invariant representations from them.
The architecture consists of DINOv2 encoder to extract the features followed by DPT decoder. At first, they train the teacher model on labelled images, and then they jointly train the student model and add in the dataset pseudo-labelled by ViT-L.
Thanks to this, Depth Anything performs very well! I have also benchmarked the inference duration of the model against different models here. I also ran torch.compile benchmarks across them and got nice speed-ups 🚀 https://huggingface2.notion.site/DPT-Benchmarks-1e516b0ba193460e865c47b3a5681efb?pvs=4
Thanks a lot for sharing these papers!
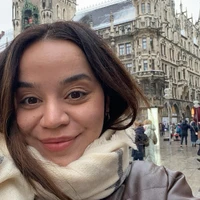
The researchers also released models fine-tuned on various downstream tasks including text categorization, NER, summarization and more! 🤯 Great models @onurgu @gokceuludogan @yirmibesogluz @furkanakkurt1618 @uskudarli 👏
Fine-tuned models are in this collection 👉 boun-tabi-LMG/turna-ft-65b3f20aff5235e6cad07c1b
Pre-trained models are in this collection 👉 boun-tabi-LMG/turna-65ad340e5df673eec66e48c7
Gotcha. Thanks a lot for sharing this 🤗 @gsarti
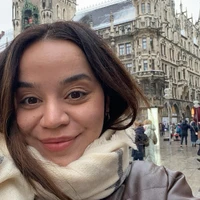
This is so cool, thanks a lot! added to my reading list :)
weights when? 😅
is it the same intuition with catastrophic forgetting?