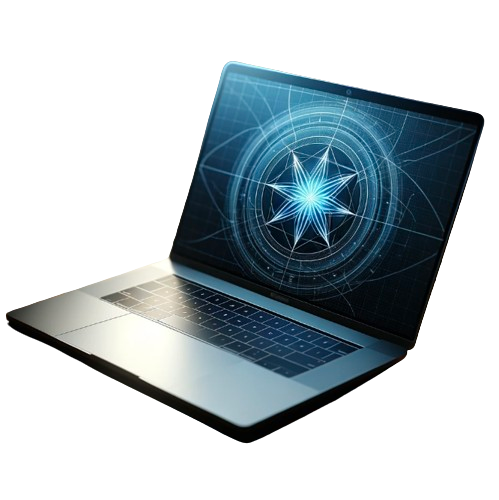
Gemma Coder π©βπ»
Gemma 2B fine-tuned on the CodeAlpaca 20k instructions dataset by using the method QLoRA with PEFT library.
Model description π§
Gemma is a family of lightweight, state-of-the-art open models from Google, built from the same research and technology used to create the Gemini models. They are text-to-text, decoder-only large language models, available in English, with open weights, pre-trained variants, and instruction-tuned variants. Gemma models are well-suited for a variety of text generation tasks, including question answering, summarization, and reasoning. Their relatively small size makes it possible to deploy them in environments with limited resources such as a laptop, desktop or your own cloud infrastructure, democratizing access to state of the art AI models and helping foster innovation for everyone.
Training and evaluation data π
CodeAlpaca_20K: contains 20K instruction-following data used for fine-tuning the Code Alpaca model.
Training hyperparameters β
Training took 1h 40 min on Free Colab T4 GPU (16GB VRAM) with the following params:
num_train_epochs=2,
per_device_train_batch_size=2,
per_device_eval_batch_size=1,
gradient_accumulation_steps=32
learning_rate=2.5e-5,
optim="paged_adamw_8bit",
logging_steps=5,
seed=66,
load_best_model_at_end=True,
save_strategy="steps",
save_steps=50,
evaluation_strategy="steps",
eval_steps=50,
save_total_limit=2,
remove_unused_columns=True,
fp16=True,
bf16=False
Training results ποΈ
Step | Training Loss | Validation Loss |
---|---|---|
50 | 1.467800 | 1.450770 |
100 | 1.060000 | 1.064840 |
150 | 0.900200 | 0.922290 |
200 | 0.848400 | 0.879911 |
250 | 0.838100 | 0.867354 |
Eval results π
WIP
Example of usage π©βπ»
I recommend install the following version of torch
:
pip install "torch>=2.1.1" -U
import torch
from transformers import AutoModelForCausalLM, AutoTokenizer, GenerationConfig
model_id = "MAISAAI/gemma-2b-coder"
tokenizer = AutoTokenizer.from_pretrained(model_id)
model = AutoModelForCausalLM.from_pretrained(model_id).to("cuda")
def generate(
instruction,
max_new_tokens=256,
temperature=0.1,
top_p=0.75,
top_k=40,
num_beams=2,
**kwargs,
):
system = f"<bos><|system|>\nYou are a helpful coding assistant.<eos>\n"
prompt = f"{system}<|user|>\n{instruction}<eos>\n<|assistant|>\n"
inputs = tokenizer(prompt, return_tensors="pt")
input_ids = inputs["input_ids"].to("cuda")
attention_mask = inputs["attention_mask"].to("cuda")
generation_config = GenerationConfig(
temperature=temperature,
top_p=top_p,
top_k=top_k,
num_beams=num_beams,
**kwargs,
)
with torch.no_grad():
generation_output = model.generate(
input_ids=input_ids,
attention_mask=attention_mask,
generation_config=generation_config,
return_dict_in_generate=True,
max_new_tokens=max_new_tokens,
early_stopping=True
)
s = generation_output.sequences[0]
output = tokenizer.decode(s, skip_special_tokens=True)
return output.split("<|assistant|>")[1]
instruction = """
Edit the following XML code to add a navigation bar to the top of a web page
<html>
<head>
<title>Maisa</title>
</head>
"""
print(generate(instruction))
Citation
@misc {maisa_ai_2024,
author = { {MAISA AI} },
title = { gemma-2b-coder (Revision e5e4e5b) },
year = 2024,
url = { https://huggingface.co/MAISAAI/gemma-2b-coder },
doi = { 10.57967/hf/2208 },
publisher = { Hugging Face }
}
- Downloads last month
- 543